Here we document all aspects of the code.
This is the multi-page printable view of this section. Click here to print.
Documentation
- 1: Overview
- 2: Installation
- 3: Docsy Documentation
- 4: Command Line Functionality
- 4.1: Input tags
- 4.2: Crystal Structure
- 4.3: General Utility for crystals
- 4.4: Symmetrizing displacements
- 4.5: Products of Irreducible Representations
- 4.6: Compute Irreducible Derivative
- 4.7: Phonon Linewidth and Thermal Conductivity
- 5: Modules
- 5.1: Command line interface
- 5.2: Translation Group
- 5.3: Symmetry
- 5.4: Representation
- 5.5: Finite Difference
- 6: Data Management
- 6.1: Data Aggregator
- 6.2: Database
- 6.3: hdf5
- 7: Unit Testing
1 - Overview
This page could probably be the landing for Overview.
What is it?
PM can be used to compute phonons and their interactions via the lone irreducible derivative (LID) and bundled irreducible derivative (BID) approaches, based on some first-principles method or using empirical potentials.
Given some anharmonic vibrational Hamiltonian, perhaps computed using PM, one can compute various vibrational observables, including phonon lineshifts and linewidths, thermal conductivity, density of states, etc.
Given some real space electronic Hamiltonian, various electronic observables can be computed, including density of states, magnetism within Hartree-Fock (assuming local interactions), etc.
Why do I want it?
Our BID algorithm for computing phonons and their interactions is the fastest finite displacement algorithm allowed by group theory.
2 - Installation
Principia Materia (PM) consists of Python codes with C++ and Fortran extensions. There will soon be a variety of options for installing PM, but we presently recommend cloning the git repository, creating a virtual environment, installing the required dependencies, and compiling the source code. We also provide the option of having auto-completion at the Bash shell using argcomplete. Please note that Python 3.7 or later is required to install and use PM.
Obtaining the source code
Clone the git repository:
git clone git@github.com:marianettigroup/principia-materia.git
Using a Virtual Environment
A virtual environment is recommended when working with PM. To setup a virtual environment:
python -m venv ENVNAME
(replace ENVNAME
with the name you choose for the environment).
To launch the environment, issue the following command:
source ENVNAME/bin/activate
This virtual environment will be launched whenever running PM.
Installing external dependencies
PM depends on several external libraries: openmp, lapack, blas, gcc, gfortran
- For Ubuntu 18.04 and later with gcc compilers
sudo apt install gcc gfortran libopenblas-dev libgomp1 libhdf5-dev
- For macOS with Homebrew
brew install gcc openblas
Required python modules for PM are listed in the file requirements.txt
,
and can be installed using pip:
Installing PM
On both Ubuntu and macOS with Homebrew, pip can be used to install python dependencies, compile fortran, and install PM. The installation step can be executed with either the regular mode:
pip install .
or in editable mode:
pip install -e .
In editable mode, the repository is dynamically link to serve as the python module so that any changes to the code come into effect without reinstalling the module. However for the extensions of the module that are written in FORTRAN, the module requires recompilation to reflect any changes to the source code. We currently recommend installing in editable mode, given that many changes are still being made to the code.
Setup Shell Auto-Complete
The auto-complete feature is optional, and is realized with the argcomplete module. To activate this feature in Bash, for example, install the module first:
pip install argcomplete
activate-global-python-argcomplete
Then within the virtual environment, source the pm-completion.sh
script in activation script ENVNAME/bin/activate
:
# append to ENVNAME/bin/activate
source ${VIRTUAL_ENV}/../pm-completion.sh
Restart the environment to activate changes.
3 - Docsy Documentation
The documentation for PM is generated using Hugo with the Docsy theme. The main advangtage of Hugo is how fast it is, and you can basically see your changes to the documentation in real time when viewing the site on a local server. The Docsy theme brings all of the advanced features that are needed for technical documentation, including detailed support for code markup (via prism), latex support (via katex), etc.
This documentation is stored in the github repository
principia-materia-docsy.
When you push to the github repository, the site will automatically be built using github actions and served at
https://marianettigroup.github.io/. If you want to push to github without rebuilding,
include [skip actions]
in your commit message.
To view the documentation locally, start by installing hugo; snap is probably the most convenient way:
sudo snap install hugo
Now change into the directory of your local pm-docsy repo, and start the hugo server:
hugo server
You can then view the docs by pointing your browser to the local port that hugo prints out, which is normally http://localhost:1313/.
If you want to add to the documentation, you will find the markdown source files in the repo here: content/en/docs/.
The command line input tags are documented in the following yaml file: data/input_tag_info.yaml. This file is automatically generated by the Data Aggregator module. There is a command line interface command which can regenerate the yaml file:
$ pm-get_variable_doc
- name: basis_atoms
description: Basis atoms of the crystal structure.
type: dict-float-array
dimension: scalar \( \leftrightarrow N_{j}\times3\) where \(j\) labels species of atom.
4 - Command Line Functionality
Most key tasks can be performed from the command line, and here we document all such functionality.
4.1 - Input tags
List of Input Tags
lattice_vectors
Testing 1 2 3
type: | real, matrix |
dimension: | 3x3 |
scripts: | xx, yy |
type: matrix
dimension: 3x3
basis_atoms
Name | Description |
---|---|
lattice_vectors | Lattice vectors in three dimensions, specified by a row-stacked 3x3 matrix. |
basis_atoms | Basis atoms of crystal structure. |
supa | A matrix of integers which left multiplies lattice_vectors to create a superlattice. |
axial_strain | A vector of three floats which specifies the axial strain. |
strain | A 3x3 real, symmetric matrix which specifies a general state of strain. |
point_group | A string specifying the point group in Schoenfiles notation. |
kpoint | A three dimensional vector specifying a kpoint. |
qpoint | A three dimensional vector specifying a qpoint. |
kpoint_mesh | A vector of three integers, specifying the kpoint mesh for electrons. |
qpoint_mesh | A vector of three integers, specifying the qpoint mesh for phonons. |
kpoint_path | A row stacked matrix of kpoints used to create a path through the BZ for plotting electronic bands. |
qpoint_path | A row stacked matrix of qpoints used to create a path through the BZ for plotting phononic bands. |
force_constants | A dictionary with keys being three dimensional vectors and values being NxN matrices, where N is the number of degrees of freedom in the unit cell. |
Tags with vector or matrix values
Many variables will be vectors or matrices, and all such variables will be processed by our text parser. Below are several examples which illustrate the variety of different ways that vectors may be input.
lattice_vectors:
[[0,0.5,0.5],
[0.5,0,0.5],
[0.5,0.5,0]]
lattice_vectors: |
0 1/2 1/2
1/2 0 1/2
1/2 1/2 0
lattice_vectors: 0 1/2 1/2; 1/2 0 1/2; 1/2 1/2 0
4.2 - Crystal Structure
For many different tasks performed in the PM suite, a crystal structure, or some component of a crystal structure, must be provided to the code. Most likely, this will be done using a yaml input file, and the two required key words are vec and atoms, which will contain the lattice vectors and the basis atoms, respectively. Considering the flourite structure, the most straightforward yaml file would be:
# xtal.yml
vec:
[[0.000000,2.764309,2.764309],
[2.764309,0.000000,2.764309],
[2.764309,2.764309,0.000000]]
atoms:
- Ca:
[[0,0,0]]
- F:
[[0.25,0.25,0.25],
[0.75,0.75,0.75]]
The code will infer that there is one basis atom labeled Ca and two basis atoms labeled F.
When entering matrices, we will always run the input through our general array parser (see parse_array if interested in the details). Therefore, there are many different options for entering a matrix as a string. For example, consider the following two examples:
scale: 2.764309
vec: |
0 1 1
1 0 1
1 1 0
atoms:
- Ca: |
0 0 0
- F: |
1/4 1/4 1/4
3/4 3/4 3/4
scale: 2.764309
vec: 0 1 1 ; 1 0 1 ; 1 1 0
atoms:
- Ca: 0 0 0
- F: 1/4 1/4 1/4 ; 3/4 3/4 3/4
where scale is a constant that multiplies all lattice vectors (a list of three constants could have been provided), and the vertical lines tells yaml to expect a text block. Our parser has a hierarchy of delimiters, ranging from space, comma, semicolon, newline. In the above example, we have two types of delimiters (i.e. spaces and newlines), which tells the code to form a matrix.
Warning
Potential Errors in yaml input
In the case of syntax error in YAML file, a RuntimeError
will be thrown indicating the an error has
occurred during the YAML loading process. For example, with the following text as YAML input:
scale: 2.764309
vec: |
0 1 1 ; 1 0 1 ; 1 1 0
atoms:
- Ca: |
0 0 0
- F: |
1/4 1/4 1/4 ; 3/4 3/4 3/4
The following error message will appear:
RuntimeError: Following error occured processing input YAML string:
ScannerError: while scanning a simple key
in "<unicode string>", line 6, column 5
could not find expected ':'
in "<unicode string>", line 7, column 3
Prototype Crystal Structures
There are a number of very common crystal structures that are adopted in numerous compounds, such as rock salt, flourite, etc. There is a simple command line script to output these crystal structures.
$ pm-prototype-xtal --perovskite
# Crystal
vec:
[[ 3.91300000, 0.00000000, 0.00000000],
[ 0.00000000, 3.91300000, 0.00000000],
[ 0.00000000, 0.00000000, 3.91300000]]
atoms:
- Sr:
[[ 0.50000000, 0.50000000, 0.50000000]]
- Ti:
[[ 0.00000000, 0.00000000, 0.00000000]]
- O:
[[ 0.50000000, 0.00000000, 0.00000000],
[ 0.00000000, 0.50000000, 0.00000000],
[ 0.00000000, 0.00000000, 0.50000000]]
4.3 - General Utility for crystals
Here we provide some of the basic functionality for periodica. A crystal structure
file must be provided on the command line, either as a filename or as standard input.
The crystal structure is simply output if no other
commands are provided. Let us use pm-prototype
to look at some examles.
# start with perovskite
pm-prototype-xtal --perovskite > xtal.yaml
pm-periodica xtal.yaml
# or we can simply do this
pm-prototype-xtal --perovskite | pm-periodica
In both cases, the output will be:
# Crystal
vec:
[[ 3.91300000, 0.00000000, 0.00000000],
[ 0.00000000, 3.91300000, 0.00000000],
[ 0.00000000, 0.00000000, 3.91300000]]
atoms:
- Sr:
[[ 0.50000000, 0.50000000, 0.50000000]]
- Ti:
[[ 0.00000000, 0.00000000, 0.00000000]]
- O:
[[ 0.50000000, 0.00000000, 0.00000000],
[ 0.00000000, 0.50000000, 0.00000000],
[ 0.00000000, 0.00000000, 0.50000000]]
Basic manipulations of the unit cell can be peformed, like shifting or permuting the atoms.
pm-periodica xtal.yaml --shift-origin 0.5,0.5,0.5 --shift-into-cell --permute-atoms 0,1,3,4,2
# Crystal
vec:
[[ 3.91300000, 0.00000000, 0.00000000],
[ 0.00000000, 3.91300000, 0.00000000],
[ 0.00000000, 0.00000000, 3.91300000]]
atoms:
- Sr:
[[ 0.00000000, 0.00000000, 0.00000000]]
- Ti:
[[ 0.50000000, 0.50000000, 0.50000000]]
- O:
[[ 0.50000000, 0.00000000, 0.50000000],
[ 0.50000000, 0.50000000, 0.00000000],
[ 0.00000000, 0.50000000, 0.50000000]]
Basic information about the lattice can be printed.
4.4 - Symmetrizing displacements
Here we illustrate how to symmtrize displacements at a given q-point according to irreducible representations of the little group using the script pm-disp-qrep. Take the example of \(\bm{q}=(\frac{1}{2},\frac{1}{2},\frac{1}{2})\) in cubic SrTiO\(_3\). We will use pm-prototype-xtal to generate the crystal structure file.
$ pm-prototype-xtal --perovskite > xtal.yml
Printing out the irreducible representations, we have
$ pm-disp-qrep xtal.yml --point-group Oh --qpoint 1/2,1/2,1/2 --print-irreps
OrbitKey(name='Sr', index=0) [0]
T2g
OrbitKey(name='Ti', index=0) [0]
T1u
OrbitKey(name='O', index=0) [0, 1, 2]
A1g+Eg+T1g+T2g
We can also print out the irreducible representation vectors.
$ pm-disp-qrep xtal.yml --point-group Oh --qpoint 1/2,1/2,1/2 --print-vectors
T2g[Sr]
0.0000 0.0000 1.0000
1.0000 0.0000 0.0000
0.0000 1.0000 0.0000
T1u[Ti]
1.0000 0.0000 0.0000
0.0000 1.0000 0.0000
0.0000 0.0000 1.0000
A1g[O]
0.5774 0.0000 0.0000 0.0000 0.5774 0.0000 0.0000 0.0000 0.5774
Eg[O]
0.7071 0.0000 0.0000 0.0000 -0.7071 0.0000 0.0000 0.0000 0.0000
-0.4082 0.0000 0.0000 0.0000 -0.4082 0.0000 0.0000 0.0000 0.8165
T1g[O]
0.0000 0.0000 0.0000 0.0000 0.0000 0.7071 0.0000 -0.7071 0.0000
0.0000 0.0000 -0.7071 0.0000 0.0000 0.0000 0.7071 0.0000 0.0000
0.0000 0.7071 0.0000 -0.7071 0.0000 0.0000 0.0000 0.0000 0.0000
T2g[O]
0.0000 0.7071 0.0000 0.7071 0.0000 0.0000 0.0000 0.0000 0.0000
0.0000 0.0000 0.0000 0.0000 0.0000 0.7071 0.0000 0.7071 0.0000
0.0000 0.0000 0.7071 0.0000 0.0000 0.0000 0.7071 0.0000 0.0000
This script will block diagonalize a dynamical matrix which has been provided. Consider the dyanmical matrix at the gamma point for STO:
$ cat dmat.yaml
dynamical_matrix_real:
3.6228 0 0 -2.990 0 0 0.2751 0 0 0.2751 0 0 -1.1822 0 0
0 3.6228 0 0 -2.990 0 0 -1.1822 0 0 0.2751 0 0 0.2751 0
0 0 3.6228 0 0 -2.990 0 0 0.2751 0 0 -1.1822 0 0 0.2751
-2.990 0 0 2.060 0 0 0.2442 0 0 0.2442 0 0 0.4416 0 0
0 -2.990 0 0 2.060 0 0 0.4416 0 0 0.2442 0 0 0.2442 0
0 0 -2.990 0 0 2.060 0 0 0.2442 0 0 0.4416 0 0 0.2442
0.2751 0 0 0.2442 0 0 4.0322 0 0 0.90 0 0 -5.460 0 0
0 -1.1822 0 0 0.4416 0 0 11.6616 0 0 -5.460 0 0 -5.460 0
0 0 0.2751 0 0 0.2442 0 0 4.0322 0 0 -5.460 0 0 0.90
0.2751 0 0 0.2442 0 0 0.90 0 0 4.0322 0 0 -5.460 0 0
0 0.2751 0 0 0.2442 0 0 -5.460 0 0 4.0322 0 0 0.90 0
0 0 -1.1822 0 0 0.4416 0 0 -5.460 0 0 11.6616 0 0 -5.4605
-1.1822 0 0 0.4416 0 0 -5.460 0 0 -5.460 0 0 11.6616 0 0
0 0.2751 0 0 0.2442 0 0 -5.460 0 0 0.90 0 0 4.0322 0
0 0 0.2751 0 0 0.2442 0 0 0.90 0 0 -5.460 0 0 4.0322
We can then block diagonalize as follows:
pm-prototype-xtal --perovskite2 | pm-disp-qrep --point-group Oh --qpoint 0,0,0 --dynamical-matrix ~/dmat.yaml
T1u-block : [('T1u', 0), ('T1u', 1), ('T1u', 2), ('T1u', 3)]
Dynamical matrix sub-block - first row
[[ 0. 0. 0. 0. ]
[ 0. 2.57 3.2 -0.18]
[ 0. 3.2 3.01 -1.33]
[ 0. -0.18 -1.33 16.7 ]]
Mass matrix sub-block - first row
[[ 36.7 5.59 -27.74 0. ]
[ 5.59 45.08 13.87 0. ]
[-27.74 13.87 69.71 0. ]
[ 0. 0. 0. 16. ]]
Mass renormalized Eigvecs
[[-0.324 0.931 -0.169 -0.017]
[ 0.692 0.111 -0.713 0. ]
[-0.644 -0.348 -0.68 -0.046]
[-0.035 0. -0.034 0.999]]
Unique Frequencies (meV)
[-8.19 -0.58 19.56 66.13]
T2u-block : [('T2u', 0)]
Dynamical matrix sub-block - first row
[[3.13]]
Mass matrix sub-block - first row
[[16.]]
Mass renormalized Eigvecs
[[1.]]
Unique Frequencies (meV)
[28.61]
It may be desirable to perform a unitary transformation within some irreducible representation subspace. Consider a yam file called unitary.yaml with the following content:
# unitary transformation to nearly diagonize the T1u subspace
- block: ['Optical',0]
irr_rep: 'T1u'
instances: [0,1]
U:
[[ -0.73059222, 0.68281403 ],
[ 0.68281403, 0.73059222 ]]
The conventional output is:
$ pm-prototype-xtal --perovskite2 | pm-disp-qrep --point-group Oh --qpoint 0,0,0 --print-vectors
('Acoustic', 0) [0, 1, 2, 3, 4]
T1u
('T1u', 0)
[[0.447 0. 0. 0.447 0. 0. 0.447 0. 0. 0.447 0. 0. 0.447 0. 0. ]
[0. 0.447 0. 0. 0.447 0. 0. 0.447 0. 0. 0.447 0. 0. 0.447 0. ]
[0. 0. 0.447 0. 0. 0.447 0. 0. 0.447 0. 0. 0.447 0. 0. 0.447]]
('Optical', 0) [0, 1, 2, 3, 4]
2T1u
('T1u', 0)
[[-0.224 0. 0. 0.894 0. 0. -0.224 0. 0. -0.224 0. 0. -0.224 0. 0. ]
[ 0. -0.224 0. 0. 0.894 0. 0. -0.224 0. 0. -0.224 0. 0. -0.224 0. ]
[ 0. 0. -0.224 0. 0. 0.894 0. 0. -0.224 0. 0. -0.224 0. 0. -0.224]]
('T1u', 1)
[[-0.866 0. 0. 0. 0. 0. 0.289 0. 0. 0.289 0. 0. 0.289 0. 0. ]
[ 0. -0.866 0. 0. 0. 0. 0. 0.289 0. 0. 0.289 0. 0. 0.289 0. ]
[ 0. 0. -0.866 0. 0. 0. 0. 0. 0.289 0. 0. 0.289 0. 0. 0.289]]
('O', 0) [2 3 4]
T1u+T2u
('T1u', 0)
[[ 0.408 0. 0. 0.408 0. 0. -0.816 0. 0. ]
[ 0. -0.816 0. 0. 0.408 0. 0. 0.408 0. ]
[ 0. 0. 0.408 0. 0. -0.816 0. 0. 0.408]]
('T2u', 0)
[[ 0. 0. 0.707 0. 0. 0. 0. 0. -0.707]
[-0.707 0. 0. 0.707 0. 0. 0. 0. 0. ]
[ 0. 0. 0. 0. -0.707 0. 0. 0.707 0. ]]
Now we can print the relevant output after the unitary transformation:
$ pm-prototype-xtal --perovskite2 | pm-disp-qrep --point-group Oh --qpoint 0,0,0 --unitary-transformation ~/unitary.yaml --print-vectors
('Optical', 0) [0, 1, 2, 3, 4]
2T1u
('T1u', 0)
[[-0.428 0. 0. -0.653 0. 0. 0.36 0. 0. 0.36 0. 0. 0.36 0. 0. ]
[ 0. -0.428 0. 0. -0.653 0. 0. 0.36 0. 0. 0.36 0. 0. 0.36 0. ]
[ 0. 0. -0.428 0. 0. -0.653 0. 0. 0.36 0. 0. 0.36 0. 0. 0.36 ]]
('T1u', 1)
[[-0.785 0. 0. 0.611 0. 0. 0.058 0. 0. 0.058 0. 0. 0.058 0. 0. ]
[ 0. -0.785 0. 0. 0.611 0. 0. 0.058 0. 0. 0.058 0. 0. 0.058 0. ]
[ 0. 0. -0.785 0. 0. 0.611 0. 0. 0.058 0. 0. 0.058 0. 0. 0.058]]
Given some distorted crystal structure, one can project the displacement onto symmetrized displacements. Consider the primitive unit cell of STO where the ferroelectric mode is allowed to condense by treating the nuclear motion as classical:
$ cat xtal_ferro.yaml
# Crystal
vec:
[[ 3.89736730, 0.00092044, 0.00092044],
[ 0.00092044, 3.89736730, 0.00092044],
[ 0.00092044, 0.00092044, 3.89736730]]
atoms:
- Sr:
[[ 0.02422827, 0.02422827, 0.02422827]]
- Ti:
[[ 0.52563170, 0.52563170, 0.52563170]]
- O:
[[ 0.51647453, 0.01719097, 0.51647453],
[ 0.51647453, 0.51647453, 0.01719097],
[ 0.01719097, 0.51647453, 0.51647453]]
Projecting onto irreducible representations gives:
pm-prototype-xtal --perovskite2 | pm-disp-qrep --point-group Oh --qpoint 0,0,0 --unitary-transformation unitary.yaml --project-xtal xtal_ferro.yaml
Projection onto irreducible representations
Block : Acoustic
----------------
T1u 0.1744 0.1744 0.1744
Block : Optical
---------------
T1u -0.0353 -0.0353 -0.0353
1.T1u -0.0018 -0.0018 -0.0018
Block : O
---------
T1u -0.0023 -0.0023 -0.0023
T2u 0 0 0
4.5 - Products of Irreducible Representations
A common group theoretical task is the direct product or symmetric direct product of irreducible representations. The composition of a product is obtained as
$ pm-irr_rep_product --point-group Oh --irr-reps T2g,T2g
# T2gxT2g = A1g+Eg+T1g+T2g
The composition of a symmetric product is obtained as
$ pm-irr_rep_product --point-group Oh --irr-reps T2g,T2g --symmetric
# [T2gxT2g] = A1g+Eg+T2g
An arbitrary number of irreps can be handled
$ pm-irr_rep_product --point-group Oh --irr-reps T2g,T2g,Eg,Eg,A2g
# T2gxT2gxEgxEgxA2g = 2A1g+2A2g+4Eg+4T1g+4T2g
Another important task is constructing the irreducible representation vectors of the product representation in terms of the rows of the original irreducible representations.
$ pm-irr_rep_product --point-group Oh --irr-reps Eg,Eg --print-irr-vectors
# EgxEg = A1g+A2g+Eg
#
# irr Eg.0xEg.0 Eg.0xEg.1 Eg.1xEg.0 Eg.1xEg.1
# A1g 0.7071 0.0 0.0 0.7071
# A2g 0.0 0.7071 -0.7071 0.0
# Eg.0 0.0 0.7071 0.7071 0.0
# Eg.1 0.7071 0.0 0.0 -0.7071
The same can be done for the symmetric product
$ pm-irr_rep_product --point-group Oh --irr-reps Eg,Eg --print-irr-vectors --symmetric
# [EgxEg] = A1g+Eg
#
# irr Eg.0xEg.0 Eg.0xEg.1 Eg.1xEg.1
# A1g 0.7071 0.0 0.7071
# Eg.0 0.0 1.0 0.0
# Eg.1 0.7071 0.0 -0.7071
It might also be useful to print out the transpose of the results, which can be done with the print-transpose flag:
$ pm-irr_rep_product --point-group Oh --irr-reps Eg,Eg --print-irr-vectors --symmetric --print-transpose
# [EgxEg] = A1g+Eg
#
# A1g Eg.0 Eg.1
# Eg.0xEg.0 0.7071 0.0000 0.7071
# Eg.0xEg.1 0.0000 1.0000 0.0000
# Eg.1xEg.1 0.7071 0.0000 -0.7071
In many cases, one is only concerned with the identity representation, and the flag only-print-identity will only print identity representations and will prune out any basis vectors that are not contained in the product:
$ pm-irr_rep_product --point-group Oh --irr-reps Eg,Eg --print-irr-vectors --symmetric --only-print-identity
# [EgxEg] = A1g+Eg
#
# Eg.0xEg.0 Eg.1xEg.1
# A1g 0.7071 0.7071
4.6 - Compute Irreducible Derivative
Within this package we can compute irreducible derivatives of phonons and phonon interactions at a given order with either LID or BID approach. The theory behind these approaches are described in the following paper:
Specifically, we can execute the LID, SS-BID and HS-BID approaches from the command line using the following commands:
pm-lid-mesh
: compute irreducible derivatives of a given FTG and order using LID method;pm-bid
: compute irreducible derivatives of a given FTG and order using SS-BID method;pm-hsbid
: compute irreducible derivatives of a given FTG and order using HD-BID method.
These commands take in generally the same command line options, therefore one can switch between approaches by changing the command while keeping
the options the same.
For example, below is guide on using the LID approach to compute irreducible derivatives of NaCl at second order but one can replace pm-lid-mesh
with pm-bid
or pm-hsbid
to execute SS-BID or HS-BID approach.
LID Approach on NaCl at Second Order
There are many ways to use this package to execute calculations of the irreducible derivatives with LID approach. Here we demonstrate a recommended workflow to execute our LID approach at second order on NaCl from forces computed by first principle method.
Symmetry Analysis and Find the Measurements to Compute
First we create a file xtal.yml
describing the structure of NaCl:
# xtal.yml
vec:
[[ 0.00000000, 2.83000729, 2.83000729],
[ 2.83000729, 0.00000000, 2.83000729],
[ 2.83000729, 2.83000729, 0.00000000]]
atoms:
- Na:
[[ 0.00000000, 0.00000000, 0.00000000]]
- Cl:
[[ 0.50000000, 0.50000000, 0.50000000]]
Then, with this structure and the point group \(O_h\), we can write the minimal configuration file, named config.yml
, to execute the LID approach with a supercell \(\hat{S}_{BZ}=2\hat1\):
# config.yml
structure: 'xtal.yml'
supa: |
2 0 0
0 2 0
0 0 2
pg: 'Oh'
order: 2
full_symmetry: True
delta: [ 0.02, 0.03, 0.04]
There are numerous parameters which have default settings that can be changed, and these can be added to the config.yml file listed above:
fdtype: 'c'
root_directory: '.'
database: 'database.db'
db_type: 'sqlite'
db_table: 'lid_phonon'
With the configuration file we can initiate the pre-processing step which will execute the symmetry analysis and create the jobs that need to be computed with first principles:
pm-lid-mesh --config config.yml --create
The above step will create two files:
lid_mesh.hdf5
: A HDF5 file containing the computed symmetry analysis results, which will be used in the post-processing step to compute the irreducible derivatives after first principle calculations are finished.database.db
: A SQLite database file containing the jobs need to be computed with first principle calculations (db_type
is specified to be ‘sqlite’ in theconfig.yml
file, other database can be used if a working interface is provided).
Note
The above steps can also be executed purely in command line with the following command:
pm-lid-mesh --save-config --create --structure xtal.yml \
--supa '2,0,0;0,2,0;0,0,2' --pg Oh --order 2 --full-symmetry \
--delta 0.02,0.03,0.04 --fdtype c --database database.db \
--db-type sqlite --db-table lid_phonon
Prepare and Execute First Principle Calculations
The next step is to create the first principle jobs to be computed, the package generically supports all types of first principle compute engines provided a working interface, and here we demonstrate using VASP. We begin by create the VASP jobs to be executed:
pm-jobs --config config.yml --create --engine vasp --save-config jobs_config.yml \
--job-config-path path-to-vasp-input-templates --root-directory jobs-ro-run
Inside the path-to-vasp-input-templates
directory, one usually needs to provide “INCAR”, “KPOINTS” and “POTCAR” files and they will be used as templates when creating the
vasp jobs, each vasp job will be created in a separate directory for convenience. Once these directories are created, VASP can be executed within each directory.
Afterwards, the calculation results can be collected and stored in the database:
pm-jobs --config jobs_config.yml --retrieve
Note
We initiated thepm-jobs
command with the input configuration file for the LID analysis config.yml
,
and subsequently saved a new configuration file jobs_config.yml
for the pm-jobs
code for the purpose of convenience.
These configuration files contains all the input arguments that are required to reproduce the exact same setup.Compute Irreducible Derivatives from the Results of First Principle Calculations
Finally, we can execute the post-processing step of the LID approach to compute the irreducible derivatives and, for example, compute the force constants tensors for Fourier interpolation:
pm-lid-mesh --load-lid-mesh lid_mesh.hdf5 --config config.yml \
--post --save-fi --save-ids --save-dts
If the material of interest is an insulator and the dipole-dipole interaction needs to be considered, the dataset for computing the dipole-dipole interaction needs to be provided as an input as well:
pm-lid-mesh --load-lid-mesh lid_mesh.hdf5 --config config.yml --epsilon path-to-epsilon.yml \
--post --save-fi --save-ids --save-dts
The dataset for computing the dipole-dipole interaction can be computed from first principle methods.
Continuing with the VASP example, we can obtain the dataset by executing a static calculation adding LEPSILON=.TRUE.
to the “ICNAR” file.
Once the calculation is finished, use the following command inside the calculation directory to parse the output from VASP and create the epsilon.yml
file:
pm-epsilon --vasp -o epsilon.yml .
then replace path-to-epsilon.yml
with the path to the generated epsilon.yml
file.
The following files are created in this step:
irreducible_derivatives.hdf5
: A HDF5 files containing the names of the irreducible derivatives and their values.fourier_interpolation.hdf5
: A HDF5 files containing all the necessary information (structure, force constants tensors, FTG and dipole-dipole contributions if at second order) to compute Fourier interpolation.dynamic_tensors.hdf5
: A HDF5 files containing all the dynamic tensors in the naive basis within irreducible Q.
Note
Both BID and HS-BID approaches can be executed following the exact same workflow while replacing the commandpm-lid-mesh
with pm-bid
and pm-hsbid
respectively, and a change of the table name for the database is also recommended (e.g. change to ‘bid_phonon’).Computing and analyzing error tails
The results of any finite displacement approach are only reliable if the discretization error has been properly controlled. Forward and backward finite difference guarantee a linear error tail, while central finite difference guarantees a quadratic error tail. Resolving the error tail is a sure sign that the discretization error has been properly extrapolated to zero. For phonons, we will be taking either the first derivatives of the forces or the second derivatives of the energy, which are typically not sensitive. Therefore, it is common practice to use a single finite displacement (i.e. a single delta), and then there is nothing to analyze. However, there are plenty of situations where one does find sensitive results, such as metallic systems with Fermi surface nesting or even band insulators when using the SCAN functional. If one computes three or more deltas, then the error tail can be scrutinized to judge the quality of the result.
To examine the error tails, the corresponding data must be output during the post-processing of results. The above lid-mesh commands needs the –err-output flag as follows:
pm-lid-mesh --load-lid-mesh lid_mesh.hdf5 --config config.yml \
--post --save-fi --save-ids --save-dts --err-output error_tails.hdf5
The next step is extract the results from the hdf5 file and output them to individual yaml files:
pm-convert-errortail error_tails.hdf5
The yaml files can then be plotted to PDF files. For example:
pm-plot-errortail 1_0_errortail.yml --iscomplex --output 1_0_errortail.pdf
At present, one can execute a loop in bash over all the files:
for i in *_errortail.yml; do
echo $i
pm-plot-errortail $i --iscomplex --output $(basename $i .yml).pdf
done
Computing phonon dispersion and DOS
With the fourier_interpolation.hdf5
file obtained at second order, we can compute phonon dispersion and density of states (DOS).
Let’s continue the NaCl example and first prepare the configuration file phonon_config.yml
for computing the phonon dispersion and DOS:
# phonon_config.yml
band:
- ['0 0 0', '1/2 1/2 0']
- ['-1/2 1/2 0', '0 0 0']
- ['0 0 0', '1/2 1/2 1/2']
band_label:
- $\Gamma$
- X
- $\Gamma$
- L
mesh: 20 20 20
Then we can execute the following command to compute the band structure and DOS:
pm-phonon-band-dos --load-fi fourier_interpolation.hdf5 --config phonon_config.yml \
--save-band --save-grid --save-dos --save-plot
The following files are created in this step:
band_plot_config.yml
: A YAML file with the configuration the plot the band structure and DOS;bands.dat
: A text file with the phonon band structure data computed with Fourier interpolation;grid_phonons.dat
: A text file with the data of the phonons that are measured at the FTG that fall on the path in the plot;dos.dat
: A text file with the data of phonon DOS.
We can plot the phonon band structure and DOS with the following command:
pm-plot-band -s band_plot_config.yml
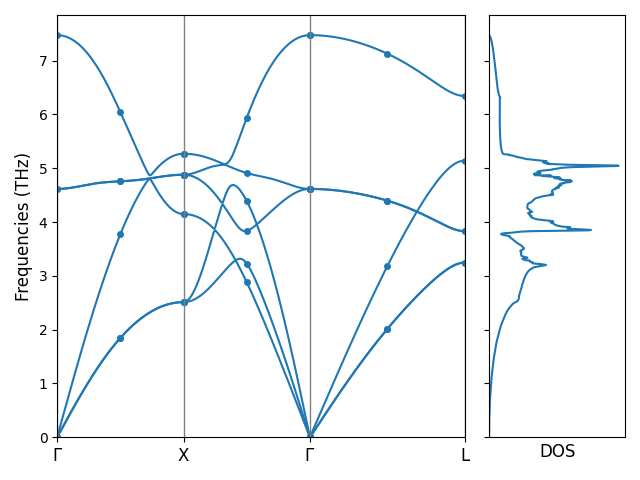
Phonon bands and DOS of NaCl
4.7 - Phonon Linewidth and Thermal Conductivity
Second and third order irreducible derivatives must be computed first, as described here, and the second and third order force constants may then be used to predict the phonon linewidth using perturbation theory. The phonons and the linewidths can then be used to computed thermal conductivity with the relaxation time approximation.
Phonon Linewidth
Here we continue the NaCl example from before.
First, we need to prepare a configuration file config.yml
for the pm-conductivity
command:
# config.yml
structure: xtal.yml
load_fi2: fi_order2.hdf5
load_fi3: fi_order3.hdf5
mesh: [11, 11, 11]
band:
- ['0 0 0', '1/2 1/2 0']
- ['-1/2 1/2 0', '0 0 0']
- ['0 0 0', '1/2 1/2 1/2']
band_label:
- $\Gamma$
- X
- $\Gamma$
- L
where:
xtal.yml
contains structure of NaCl;fi_order2.hdf5
is the HDF5 data file for the FourierInterpolation class at second order, which contains the 2nd order force constants;fi_order3.hdf5
is the HDF5 data file for the FourierInterpolation class at third order, which contains the 3rd order force constants.
In this example, we use the FourierInterpolation object of a \(\hat{S}_{BZ}=4\hat{\mathbf{1}}\) FTG (i.e. a \(4 \times 4 \times 4\) supercell) at second order and the FourierInterpolation object of a \(\hat{S} _{BZ}=2\hat{\mathbf{1}}\) FTG (i.e. a \(2 \times 2 \times 2\) supercell) at third order. We interpolate the phonons and phonon interactions to the mesh of \(11\hat{\mathbf{1}}\), and compute the linewidth along the K-path \(\Gamma \to X \to \Gamma \to L\).
Execute the following command the compute the phonon linewidth:
pm-conductivity --config config.yml --save-gamma
Thermal Conductivity
Using the same NaCl example, we can prepare a configuration file config.yml
similar to the one above:
# config.yml
structure: xtal.yml
load_fi2: fi_order2.hdf5
load_fi3: fi_order3.hdf5
mesh: [11, 11, 11]
Execute the following command the compute the thermal conductivity using relaxation time approximation (RTA):
pm-conductivity --config config.yml --save-kappa --mode RTA
5 - Modules
Here we document various classes and functions within each module, and provide minimal examples.
5.1 - Command line interface
All command line script for PM are stored in the cli
module, end with the suffix _cli
,
and are a class named CommandLine
which derives from BaseCommandLine
.
In order to register a new cli, the script must be added to
the setup.cfg
file. Consider the example irr_rep_prod_cli.py
: there is
an entry in the console_scripts
# setup.cfg file
console_scripts =
pm-irr_rep_product = principia_materia.cli.irr_rep_product_cli:CommandLine.main
After updating the file, you must run pip installi .
or pip install -e .
to make
the changes take effect. You also may need to restart your virtual environment for
tab completion to be updated.
5.2 - Translation Group
When performing calculations, we need to use various properties of both the infinite translation group (Lattice) and the finite translation group (LatticeFTG). The infinite translation group contains the lattice vectors, and computes various information from them (e.g. vector lengths and angles, volume, reciprocal lattice, etc). The FTG is naturally characterized by a supercell \(\hat{S}_{BZ}\), and LatticeFTG derives from Lattice. Finding all translation points within a given supercell is a key task. Similarly, another key task is finding all the k-points commensurate with a given FTG, but this is currently execute in the separate kpoints class.
Additionally, we will need to find the smallest supercell that will accomodate a given set of \(\bm q\)-points.
Lattice
Here introduce the basic functionality of the Lattice
class using the
face center cubic as a demonstration:
>>> import numpy as np
>>> from principia_materia.translation_group import Lattice
>>> fcc_vec = 0.5*(np.ones((3,3))-np.identity(3))
>>> lattice = Lattice(vec=fcc_vec)
>>> lattice.vec
array([[0. , 0.5, 0.5],
[0.5, 0. , 0.5],
[0.5, 0.5, 0. ]])
With the above Lattice class object, we can compute various properties of the lattice.
>>> print("volume:", lattice.vol)
volume: 0.25
>>> print("lengths of the lattice vectors:", lattice.abc)
lengths of the lattice vectors: [0.70710678 0.70710678 0.70710678]
>>> print("angles between the lattice vectors:", lattice.abg)
angles between the lattice vectors: [60. 60. 60.]
There is a reciprocal lattice associated with the lattice, which is also constructed in this class, and we follow the convention \(\bm a_i\cdot \bm b_j =2\pi \delta_{ij} \), where \(\bm a_i\) is a real space vector and \(\bm b_j\) is a reciprocal space vector.
>>> print(lattice.rvec)
[[-6.28318531 6.28318531 6.28318531]
[ 6.28318531 -6.28318531 6.28318531]
[ 6.28318531 6.28318531 -6.28318531]]
>>> print("volume of reciprocal lattice:", lattice.rvol)
volume of reciprocal lattice: 992.200854
>>> print("lengths of the reciprocal lattice vectors:", lattice.rabc)
lengths of the reciprocal lattice vectors: [10.88279619 10.88279619 10.88279619]
>>> print("angles between the reciprocal lattice vectors:", lattice.rabg)
angles between the reciprocal lattice vectors: [109.47122063 109.47122063 109.47122063]
Additionally, the Lattice class allows us to perform various operation to a lattice, for example applying an axial strain:
>>> strain = [0.02, 0, 0] # axial strain along x
>>> example_lattice = lattice.copy()
>>> example_lattice.axial_strain(strain)
>>> print(example_lattice.vec)
[[0. 0.5 0.5 ]
[0.51 0. 0.5 ]
[0.51 0.5 0. ]]
LatticeFTG
LatticeFTG is the class which handles finite translation groups, and the key method of the class finds all tranlsation points (i.e. t-points) contained within the supercell. While this result can be obtained from a simple Cartesian product in the case of a diagonal supercell, non-diagonal supercells are more subtle. Non-diagonal supercells are important in the context of lattice dynamic, because it is always preferrable to work with the smallest number of irreducible derivatives which yield a smooth interpolation, and non-diagonal supercells yield multiplicities which cannot be obtained solely using diagonal supercells. Therefore, it is important to have a robust algorithm to find all lattice points within an arbitrary supercell, and our algorithm is detailed in Appendix D in PRB 100 014303. The same algorithm can be reversed to compute the indices of the lattice points at \(\mathcal{O}(1)\) time complexity, removing the need to create a hash function.
Below is a simple example which is easily recognizable: a fcc lattice with a supercell that yields the conventional cubic cell.
>>> import numpy as np
>>> from principia_materia.translation_group import LatticeFTG
>>> fcc_vec = 0.5*(np.ones((3,3))-np.identity(3))
>>> S_bz = np.ones((3, 3)) - 2 * np.identity(3)
>>> lattice_ftg = LatticeFTG(fcc_vec,S_bz)
>>> lattice_ftg.vec
array([[0. , 0.5, 0.5],
[0.5, 0. , 0.5],
[0.5, 0.5, 0. ]])
>>> lattice_ftg.supa
array([[-1, 1, 1],
[ 1, -1, 1],
[ 1, 1, -1]])
>>> lattice_ftg.tpoints
array([[0, 0, 0],
[0, 0, 1],
[0, 1, 0],
[1, 0, 0]])
>>> lattice_ftg.get_index(lattice_ftg.tpoints)
array([0, 1, 2, 3])
>>> lattice_ftg.supa_vec
array([[1., 0., 0.],
[0., 1., 0.],
[0., 0., 1.]])
>>> lattice_ftg.supa_tpoints
array([[ 0 , 0 , 0 ],
[1/2, 1/2, 0 ],
[1/2, 0 , 1/2],
[ 0 , 1/2, 1/2]], dtype=object)
KpointsFTG
We have a dedicated class for construcint k-points, though this is basically just a trivial wrapper around LatticeFTG.
>>> import numpy as np
>>> from principia_materia.translation_group import KpointsFTG
>>> fcc_vec = 0.5*(np.ones((3,3))-np.identity(3))
>>> S_bz = np.ones((3, 3)) - 2 * np.identity(3)
>>> qpts = KpointsFTG(vec=fcc_vec, supa=S_bz)
>>> qpts.ppoints
array([[0, 0, 0],
[0, 0, 1],
[0, 1, 0],
[1, 0, 0]])
>>> qpts.kpoints
array([[ 0 , 0 , 0 ],
[1/2, 1/2, 0 ],
[1/2, 0 , 1/2],
[ 0 , 1/2, 1/2]], dtype=object)
>>> qpts.ppoints_to_kpoints(
... np.array([[0, 0, 1],
... [1, 0, 0]]) )
array([[1/2, 1/2, 0 ],
[ 0 , 1/2, 1/2]], dtype=object)
>>> qpts.kpoints_to_ppoints(
... np.array([[0.5, 0.5, 0 ],
... [0 , 0.5, 0.5]]) )
array([[0, 0, 1],
[1, 0, 0]])
KpointsFSG
Similar to KpointsFTG, the class KpointsFSG is basically a trivial wrapper around LatticeFSG, which handles the point symmetry of the lattice. Given that the most common use cases of point symmetry are in reciprocal space, only KpointsFSG is outlined here. KpointsFSG derives from KpointsFTG, so it shares all the same attributes outlined above.
>>> import numpy as np
>>> from principia_materia.translation_group import KpointsFSG
>>> fcc_vec = 0.5*(np.ones((3,3))-np.identity(3))
>>> S_bz = 2*np.identity(3)
>>> qpts = KpointsFSG(vec=fcc_vec, supa=S_bz, pg='Oh')
>>> qpts.kpoints
array([[ 0 , 0 , 0 ],
[1/2, 0 , 0 ],
[ 0 , 1/2, 0 ],
[1/2, 1/2, 0 ],
[ 0 , 0 , 1/2],
[1/2, 0 , 1/2],
[ 0 , 1/2, 1/2],
[1/2, 1/2, 1/2]], dtype=object)
>>> qpts.irr_kpoints
array([[ 0 , 0 , 0 ],
[1/2, 0 , 0 ],
[1/2, 1/2, 0 ]], dtype=object)
>>> qpts.irr_ppoints
array([[0, 0, 0],
[1, 0, 0],
[1, 1, 0]])
>>> qpts.N_irr
3
>>> qpts.irr_indexes
array([0, 1, 3])
>>> qpts.indexes_map_irr_index
array([0, 1, 1, 3, 1, 3, 3, 1])
>>> qpts.indexes_map_irr_order
array([0, 1, 1, 2, 1, 2, 2, 1])
>>> qpts.stars
[[0], [1, 2, 4, 7], [3, 5, 6]]
>>> qpts.little_groups
['Oh', 'D3d', 'D4h']
>>> qpts.kpoint_type
['real', 'real', 'real']
>>> # operations that yield 4th point from irr counterpart
>>> qpts.indexes_map_irr_ops[4]
['c3be', 'ci3de', 'c2y', 'c4x', 'c4z', 'c2d', 'Ic3be', 'Ici3de', 'Ic2y', 'Ic4x', 'Ic4z', 'Ic2d']
QpointsN
Next, we can use the above information to find irreducible \(Q\)-points.
>>> qpointsn = QpointsN(vec=lattice_vectors, supa=supa, order=3, pg=pg)
>>> qpointsn.find_irreducible_Qpoints()
array([[[Fraction(0, 1), Fraction(0, 1), Fraction(0, 1)],
[Fraction(0, 1), Fraction(0, 1), Fraction(0, 1)],
[Fraction(0, 1), Fraction(0, 1), Fraction(0, 1)]],
...,
[[Fraction(3, 4), Fraction(1, 4), Fraction(1, 2)],
[Fraction(3, 4), Fraction(1, 4), Fraction(1, 2)],
[Fraction(1, 2), Fraction(1, 2), Fraction(0, 1)]]], dtype=object)
get_minimum_supercell
Algorithm to find the minimum supercell for a given list of \(\textbf{q}\)-points
The minimum supercell multiplicity is derived in the paper. Here using the implemented function, we find that for the \(\textbf{q}\)-point \(\textbf{q}=\left(\frac{1}{4}, \frac{3}{4}, 0\right)\) the supercell of the minimum multiplicity is:
$$ \hat{S}_{\textbf{q}} = \begin{bmatrix} 1 & 1 & 0 \\ 0 & 4 & 0 \\ 0 & 0 & 1 \end{bmatrix} $$
, and for the \(Q\)-point \(Q=\left[\left(\frac{1}{4}, \frac{3}{4}, 0\right), \left(\frac{1}{4}, \frac{1}{2}, 0\right), \left(\frac{3}{4}, \frac{1}{4}, \frac{1}{2}\right)\right]\) the supercell of the minimum multiplicity is:
$$ \hat{S}_{Q} = \begin{bmatrix} 4 & 0 & 0 \\ 0 & 4 & 0 \\ 0 & 0 & 2 \end{bmatrix} $$
>>> import numpy as np
>>> from principia_materia.translation_group import get_minimum_supercell
>>> from principia_materia.io_interface import parse_array
>>> from principia_materia.mathematics import Fraction
>>> Qpoint = parse_array("""\
... 1/4 3/4 0
... """, dtype=Fraction)
>>> Qpoint
array([1/4, 3/4, 0 ], dtype=object)
>>> get_minimum_supercell(Qpoint)
array([[1, 1, 0],
[0, 4, 0],
[0, 0, 1]])
>>> Qpoint = parse_array("""\
... 1/4 3/4 0
... 1/4 1/2 0
... 3/4 1/4 1/2
... """, dtype=Fraction)
>>> Qpoint
array([[1/4, 3/4, 0 ],
[1/4, 1/2, 0 ],
[3/4, 1/4, 1/2]], dtype=object)
>>> get_minimum_supercell(Qpoint)
array([[4, 0, 0],
[0, 4, 0],
[0, 0, 2]])
5.3 - Symmetry
Here we encode all information about the crystallographic point groups. In the near future, we will add space group information here as well.
Cornwell_group_matrices
Cornwell_point_group
PointGroup
get_little_group
5.4 - Representation
This module contains all classes relevant to building a representation of a group, finding the composition in terms of irreducible representations, and building irreducible vectors.
BaseRepresentation
CharmBlochRep
ShiftMode
ClusterRep
DispClusterRep
SingleTensorRep
DirectProduct
SymmetricDirectProduct
StarCharmBlochRep and Stars
ProductofQIrreps
SymmetricProductofQIrreps
QStarRep
SymmetricQStarRep
5.5 - Finite Difference
Finite difference is used to compute phonons and phonon interactions in PM, and this module isolates all the tasks associated with performing finite difference. Here we give some examples to illustrate the key features.
Let us consider a potential with three variables, labeled 0, 1, 2, and set up all derivatives to second order. Begin by instantiating the class:
>>> from principia_materia.finite_difference.finite_difference_np import FiniteDifferenceNP
>>> fd=FiniteDifferenceNP()
Set all the derivatives to be evaluated:
>>> fd.set_deriv(varset=[[0],[1],[2],[0,1],[0,2],[1,2]],
... ordset=[[2],[2],[2],[1,1],[1,1],[1,1]])
Set the deltas to be the same for all variables and setup the finite difference amplitudes:
>>> fd.set_delta_global([0.01,0.02])
>>> fd.setup_amplitude()
We now have all the information we need to perform finite difference. For example, all amplitudes associated with a given set of variables is retrieved as:
>>> print (fd.get_var_amplitude([0,2]))
[[-0.02 -0.02]
[-0.02 0.02]
[-0.01 -0.01]
[-0.01 0.01]
[ 0.01 -0.01]
[ 0.01 0.01]
[ 0.02 -0.02]
[ 0.02 0.02]]
In more complicated scenarios, the same amplitudes would appear multiple times, but this class accounts for that when making a final list of amplitudes. Take the following example containing second and fourth derivatives:
>>> fd.set_deriv(varset=[[0,1],[0,1]],
... ordset=[[1,1],[2,2]])
Inspecting the list of amplitudes for variable [0,1]
, we see:
>>> print (fd.get_var_amplitude([0,1]))
[[-0.02 -0.02]
[-0.02 0.02]
[-0.01 -0.01]
[-0.01 0.01]
[ 0.01 -0.01]
[ 0.01 0.01]
[ 0.02 -0.02]
[ 0.02 0.02]
[-0.04 -0.04]
[-0.04 0.04]
[ 0.04 -0.04]
[ 0.04 0.04]]
The same amplitudes are used for both the second and fourth derivatives, and
the class is aware of this. Additionally, the fourth
derivatives will need cases where one amplitude is zero, which do not appear.
In this case, the class creates a new variable sets [0]
and [1]
that contain
these amplitudes. For example,
>>> print (fd.get_var_amplitude([0]))
[[-0.04]
[-0.02]
[ 0.02]
[ 0.04]]
6 - Data Management
PM deals with varied data sets, some of which is read in from other sources and some of which is generated.
6.1 - Data Aggregator
Like many python-based application, PM will need to take input data from the command line and or YAML input files. A mechanism is needed to characterize the data, enforce the data type of the input data, and possibly do some preprocessing of the data. Data Aggregator (DA) handles these tasks. DA uses descriptors to encode the properties of a collection of data.
Descriptors
Input data is characterized by descriptors. A descriptor describes a set of data associated with some entity, which is normally a class or a script. The descriptor will normally be named by the class or command line interface they correspond to. Below we document the general structure of a descriptor named descriptor_name.
descriptor_name:
type: generic # allowed values are generic, class, or script
description:
A description of the collection of variables. If argparse
is used, this will be passed on.
inherit:
- descriptor1 # inherit properties of descriptor1
- descriptor2 # inherit properties of descriptor1
conflicts:
- [var1, var2] # var1 and var2 cannot both be specified
- [var1, var3, var4] # var1, var3, var4 cannot all be specified
variables:
var1:
type: int # a full list of data type provided elsewhere
optional: True # var1 is not required; default is False
dimension: scalar # this is only for PM documentation
code_alias: v1 # sometimes an alias is used within code
alias:
- variable1 # multiple alias can be given for argparse
choices: # var1 can only have values of 1 or 2
- 1
- 2
requires:
- var5 # if var1 is provided, var5 must be given too
default: 5.2 # a default value may be provided
help:
A description of the variable.
Using DA to parse and enforce data types
One degree of functionality for DA is to apply data types to input data. Consider a minimal example of a simple script, where one might have some data in a yaml file that is read in:
import yaml
from principia_materia.io_interface.data_aggregator import DataAggregator
descriptor = yaml.safe_load("""
script_input:
description:
An example of a descriptor.
variables:
lattice_vectors:
type: float-array
scale:
type: float
help: a factor used to rescale the lattice vectors.
""")
data = yaml.safe_load("""
lattice_vectors: |
0 1 1
1 0 1
1 1 0
scale: 4.8
""")
data_ag = DataAggregator(
'script_input',
descriptor_definition = descriptor,
input_data = data)
print (data_ag.data)
# {'lattice_vectors': array([[0., 1., 1.],
# [1., 0., 1.],
# [1., 1., 0.]]), 'scale': 4.8}
print (data_ag.data_tuple)
# script_input(lattice_vectors=array([[0., 1., 1.],
# [1., 0., 1.],
# [1., 1., 0.]]), scale=4.8)
DA uses the parse_array
function to parse strings into arrays, which is
documented elsewhere.
Using DA with argparse
DA descriptors contain all the information needed to construct an argparse instance which can be used to get information from the command line. We continue the previous example, but now instead of obtaining data by definition, we will get all variables from the command line. The call to DataAggregator will now not provide any data, but instead provides instructions to use argparse, which is the default behavior.
data_ag = DataAggregator(
'script_input',
descriptor_definition = descriptor,
use_argparse=True)
The default of use_argparse
is True, so this variable only needs to be specified
if one does not want the overhead of instantiating argparse.
We can then call the script, called test_script.py
, from the command line:
$ test_script.py --lattice-vectors '0 1 1 , 1 0 1 , 1 1 0' --scale 4.8
It should be emphasized that one can both provide input data and use argparse, and by default argparse takes presidence; allowing one to easily override variables from the command line.
All of the data from the descriptor has been loaded into argparse, and
issuing the help flag -h
or --help
will generate the following:
$ test_script.py -h
# usage: test.py [-h] [--lattice-vectors LATTICE_VECTORS] [--scale SCALE]
#
# An example of a descriptor.
#
# options:
# -h, --help show this help message and exit
# --lattice-vectors LATTICE_VECTORS
# --scale SCALE a factor used to rescale the lattice vectors.
Using DA with input files
We have now considered directly taking input from yaml string and taking input from the command line via argparse. Given that a common use case will be to take yaml input from some yaml file, DA allows one to specify a variable name which will take in a list of strings which are names of yaml files which are meant to be processed. Consider taking our input and breaking it into two yaml files:
# file1.yaml
lattice_vectors: |
0 1 1
1 0 1
1 1 0
# file2.yaml
scale: 4.8
Now we can tell DA that there will be a variable that will contain input file
names using the input_files_tag='input_files'
variable. Additionally, we will
need to add this variable to our descriptor:
descriptor['script_input']['variables']['input_files']={
'optional':True,
'type': 'string-list'
}
data_ag = DataAggregator(
'script_input',
descriptor_definition = descriptor,
input_files_tag = 'input_files'
use_argparse = True)
We can then call the script:
$ test_script.py --inputs-files file1.yaml,file2.yaml
By default, we have input_files_tag = 'input_files'
and use_argparse = True
, so
these variables do not need to be specified.
Using DA with a required input file
In addition to specifying input files as described above, it is possible to also specify
a required input file, which demands that an input file must be given on the command line
or piped into standard input. The is specified in the constructor using require_file=True
.
This would be desirable if there is a script that requires enough data input that an input
file will always be used, allowing the user to specify the file name but not use any flags.
For example, we could have
# file1.yaml
lattice_vectors: |
0 1 1
1 0 1
1 1 0
scale: 4.8
and then any of the three commands could be used:
$ test_script.py file1.yaml
$ test_script.py < file1.yaml
$ cat file1.yaml | test_script.py
Setting data heirarchy in DA
It should be emphasized that one
can simultaneously specify variables through the constructor, the command line,
input files, and a required input file, allowing data to be taken from four different sources.
The precedent for each source of data is specified by the hierarchy=[0,1,2,3]
variable in
the constructor, where the number given in the list corresponds to the following data sources:
data_sources = [ input_file_data, # data from input yaml files
required_file_data, # data from a required file
args_data, # data from command line
input_data ] # data supplied through constructor
The hierarchy variable starts with lowest priority and ends with highest priority, which means that the default behavior is that regular input files have lowest priority and data given in the constructor has the highest priority.
Pre-defined descriptors
DA will look for a file called descriptors.yaml
in the same directory where data_aggregator.py
is located. Descriptors have been created for many classes and command line interfaces in PM.
Instantiating classes using DA
DA can be used to instantiate a class using the aggregated data. Continuing with the above example, we could instantiate the Lattice class, where the constructor requires lattice_vectors.
from principia_materia.translation_group.lattice import Lattice
lattice = data_ag.get_instance(Lattice)
DA will pass all variables that Lattice allows, and withhold the rest.
6.2 - Database
6.3 - hdf5
7 - Unit Testing
Here we document how unit testing is performed for PM, and provide an overview of what sort of tests are performed. Unit tests for PM are stored in a separate repository called principia-materia-tests.
PM performs unit testing with Pytest, which is a standard framework for unit testing within python. All unit tests may be run by going into the repo, installing the required dependencies, and executing pytest
.
git clone git@github.com:marianettigroup/principia-materia-tests.git
cd principia-materia-test
pip install requirements.txt
pytest
An individual test can be run by changing into a directory and running pytest on a specific test. For example,
cd tests/translation_group
pytest test_translation_group.py